A good rule of thumb when entering a casino is that if you are offered action on anything, then your most profitable choice is to walk away. Everything offered in a casino is designed to take your money. Even the stuff not actually offered by the casino itself.
A friend of mine, let's call him Mike, told me a story of a time he went to a casino to casually play some poker (Texas Hold'em to be exact). While at the table, he struck up a conversation with the guy sitting next to him. He seemed friendly enough, although possibly a complete sucker. The man insisted that 2s, 7s, and Jacks are the luckiest cards in the deck. While not a professional gambler or even a mathematician, Mike was smart enough to know how ridiculous that claim was on its face. After all, there are 52 cards in the deck and 13 different ranks. How could 3 of those ranks be luckier than the other 10?
The man then proposed a bet: anytime a 2, 7, or J appeared on the flop (the flop is the first three community cards dealt), then he won. If none of those cards appeared, then Mike won. Mike felt this was too good to be true, and thus he agreed to keep this bet going for every flop seen on the table at $5 per hand.
Two hours later, Mike was down $50 in the side bet (not to mention the rest of his money from the actual poker game) and called it a night. Does it turn out 2s, 7s, and Js really are the luckiest cards in the deck after all?

Nope, not even in the slightest! It turns out that Mike was right that the man's claim about lucky cards was as ridiculous as it sounded, but his conviction caused him to be misdirected into an old carny's game. We can use basic probability and statistics to prove that.
Let p = the probability that none of the three "lucky" ranks (2, 7, or J) appears on any of the flop cards. There's 52 cards in the deck, 3 on each flop, and 40 of them are not a 2/7/J. If three non-lucky cards were flopped, then...
p = (40/52) * (39/51) * (38/50) = .447%
Alternatively, you could write this as the combination of 40 choose 3 divided by 52 choose 3 to get the same answer. This counts the fraction of possible three non-lucky card flops over the number of all possible three card flops.
So if p is 44.7%, then the opposite of p (or the probability that at least one "lucky" card is flopped) equals 1 - p, which is 55.3%. This means that Mike was giving up a 10.6% edge. By comparison, the house edge in roulette, one of the casino's most profitable table games, is almost exactly half of that, 5.26%. Even the most clueless blackjack player taking actions totally at random is estimated to only be giving up about a 3% edge to the house. Yet somehow Mike has found the casino game where he's giving up 10.6%, and he's giving it up to the guy who he thought was the real sucker!
What happened!?!? Mike correctly identified the imbalance of non-lucky cards to lucky cards, but he failed to take into account the repetition. Remember that there are three cards on each flop. So even if a lucky card is an underdog to appear on any given card, the odds swing way more in its favor to come through as the draw gets repeated. A similar example is the Jets ongoing quest to go 0-16. My latest simulation gives the Jets only a 19% chance of losing their last 7 games. That may sound very low considering they will be a heavy underdog in each game, but underdogs are more likely to come through the more chances you give them.
Poor Mike now knows he took a bad bet, but he swears he was still unlucky in the game to have lost $50 (just like any true gambler, blaming all of his losses on luck alone). The good news is that we can use statistics to prove that as well. Let us estimate that a live poker table sees 30 flops per hour. They played for two hours which equals 60 flops, and at $5 per flop then they would have bet $300 in handle.
Mean expected loss = $300 * .106 = $31.80
n = 60 flops
p = .447
1 - p = .553
Standard deviation = √[(p)*(1-p) / n] = .0642
I simulated the distribution 50,000 times, as seen below. I also overlaid where the mean loss, actual loss, and breakeven point were located.
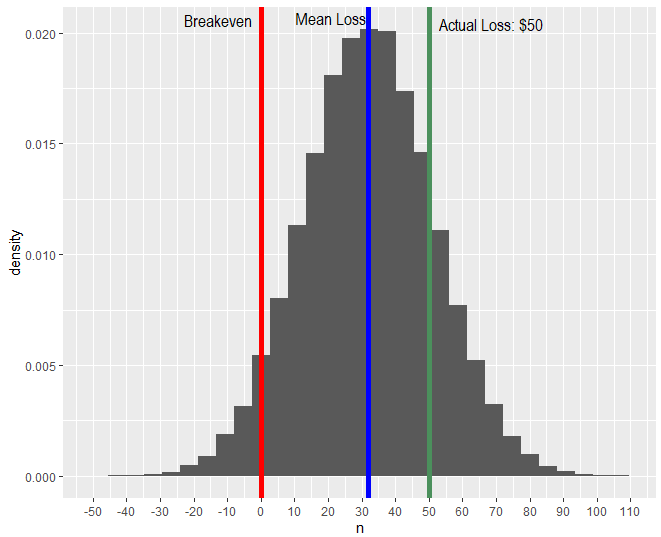
Mike's loss of $50 was in the 82nd percentile of simulations. While that was on the negative side of expectation, it was ultimately less than 1 standard deviation away from the mean expected loss. Unlucky but not abnormally so, especially when you consider he only had a 4.8% chance of actually breaking even in this game to begin with. Consider that if Mike had been running about 1 standard deviation above expectation instead of below, then he still would have lost $10. In other words, Mike could've been a lot luckier and still probably would have ended up losing. Mike's loss was not just from bad luck; it was from bad game selection.
Sorry, Mike, but I believe Matt Damon's character in Rounders said it best:

Commenti